Искусственный интеллект преобразует современные производственные линии способами, которые мы когда-то только могли себе представить. В этой статье объясняется, как системы инспекции ИИ оптимизируют процессы упаковки, минимизируя отходы. Если вас волнуют эффективность производства, безопасность пищевых продуктов и экологичные решения, продолжайте читать. Представленные здесь идеи могут помочь вам принять более разумное и экологичное будущее.
Почему стоит сосредоточиться на контроле качества упаковки с помощью искусственного интеллекта?
Упаковка в виде пакета чрезвычайно популярна для закусок, жидкостей, корма для животных и многих других продуктов. Она экономит место на полках, легко открывается и обеспечивает привлекательный вид. По моему опыту, использование Инспекция с помощью искусственного интеллекта для упаковки пакетов обеспечивает новый уровень точности. Автоматизированные камеры проверяют каждый пакет в режиме реального времени, помогая убедиться, что каждый шов герметичен и надежен. Если пакет не заполнен или имеет небольшой дефект, система может мгновенно пометить его на станция отбраковки и не дать ему двигаться дальше.
Один важный термин, который следует иметь в виду: целостность уплотнения, что означает прочность и качество уплотнения упаковки. Традиционные методы основаны на отборе проб и визуальном контроле людьми, что может быть медленным и подверженным ошибкам. Системы на основе ИИ анализируют микродетали, которые невооруженный глаз может пропустить, например натяжение пленки или крошечные морщины в пластике. Это помогает гарантировать, что потребители получат нужное количество продукта, без риска протечек или загрязнения.
«Качество никогда не бывает случайностью; оно всегда является результатом разумных усилий». — Джон Раскин
Однажды я экспериментировал с установкой встроенного устройства ИИ-видения на линии наполнения пакетов. Разница была как день и ночь. Машина быстро обнаруживала дефекты еще до окончательной герметизации, что экономило время и материалы.
Как ИИ-упаковка меняет производство?
Упаковка ИИ меняет то, как мы создаем, заполняем и распространяем продукцию. Традиционные производственные линии полагаются на фиксированные датчики, которые определяют наличие объекта, но не более того. Напротив, усовершенствованные датчики на базе искусственного интеллекта распознают тонкие закономерности в форме, цвете или размере. Эти умные датчики запускать корректирующие действия за считанные секунды.
Критерии | Традиционные упаковочные линии | Упаковочные линии с искусственным интеллектом |
---|---|---|
Метод проверки | Ручная или базовая проверка датчиков | Проверка зрения с помощью алгоритмов ИИ |
Скорость обнаружения ошибок | Более высокий уровень упущенных недостатков | Точность обнаружения более 99% во многих случаях |
Подход к обслуживанию | Реактивный (ремонт после поломки) | Предиктивный (исправление до возникновения сбоя) |
Использование материалов | Часто чрезмерный из-за менее точного контроля | Оптимизировано для сокращения отходов |
Масштабируемость | Ограниченная гибкость; необходимы серьезные обновления | Адаптивный и легко масштабируемый через обновления программного обеспечения |
Согласие | Опирается на ручные проверки соблюдения правил | Автоматизированное отслеживание пломб, маркировки и посторонних предметов |
Сбор данных | Минимальные или ручные журналы | Комплексные данные в реальном времени хранится в цифровом формате |
Одним из примеров является система непрерывной подачи: упаковочная пленка подается на линию без остановки, и машина сканирует пленку на предмет любых неровностей, таких как разрывы, несоосность или пятна чернил. ИИ может даже измерять точность цветопередачи на этикетках или фирменных изображениях, предупреждая операторов, если что-то не так.
Этот высокий уровень машинного интеллекта также помогает в сокращение отходов. Раннее обнаружение дефектов позволяет тратить меньше материалов на бракованную упаковку. Во время пилотного запуска я наблюдал сокращение отходов пленки и пластика 25% после запуска проверки ИИ. Производители, которые хотят достичь растущих целей в области устойчивого развития, получат большую выгоду от этих улучшений.
Упаковочная машина с контролем качества на основе искусственного интеллекта
А Упаковочная машина с контролем качества на основе искусственного интеллекта часто поставляется с необходимыми функциями:
- Распознавание изображений в реальном времени
Высокоскоростные камеры фиксируют каждый шаг, делая сотни изображений в минуту. Распознавание изображений Затем алгоритмы обрабатывают данные, выявляя искажения формы или цвета. - Адаптивные алгоритмы
Алгоритмы ИИ обучаются на каждом новом производственном цикле, корректируя такие параметры, как натяжение или давление. Эта адаптивная природа позволяет системе быстро реагировать при появлении любого дефекта. - Интеграция метаданных
Современные машины интегрируются с существующими корпоративными системами, сохраняя важные данные о номерах партий, скорости производства и условиях окружающей среды.
Из моего собственного практического опыта, самое большое преимущество — это уверенность, которую она приносит. Система профилактическое обслуживание Модуль отправляет оповещения, если что-то вот-вот выйдет из строя, сокращая время простоя. Операторы также могут отслеживать статистику упаковочной линии в режиме реального времени, что упрощает планирование использования ресурсов или графиков смен.
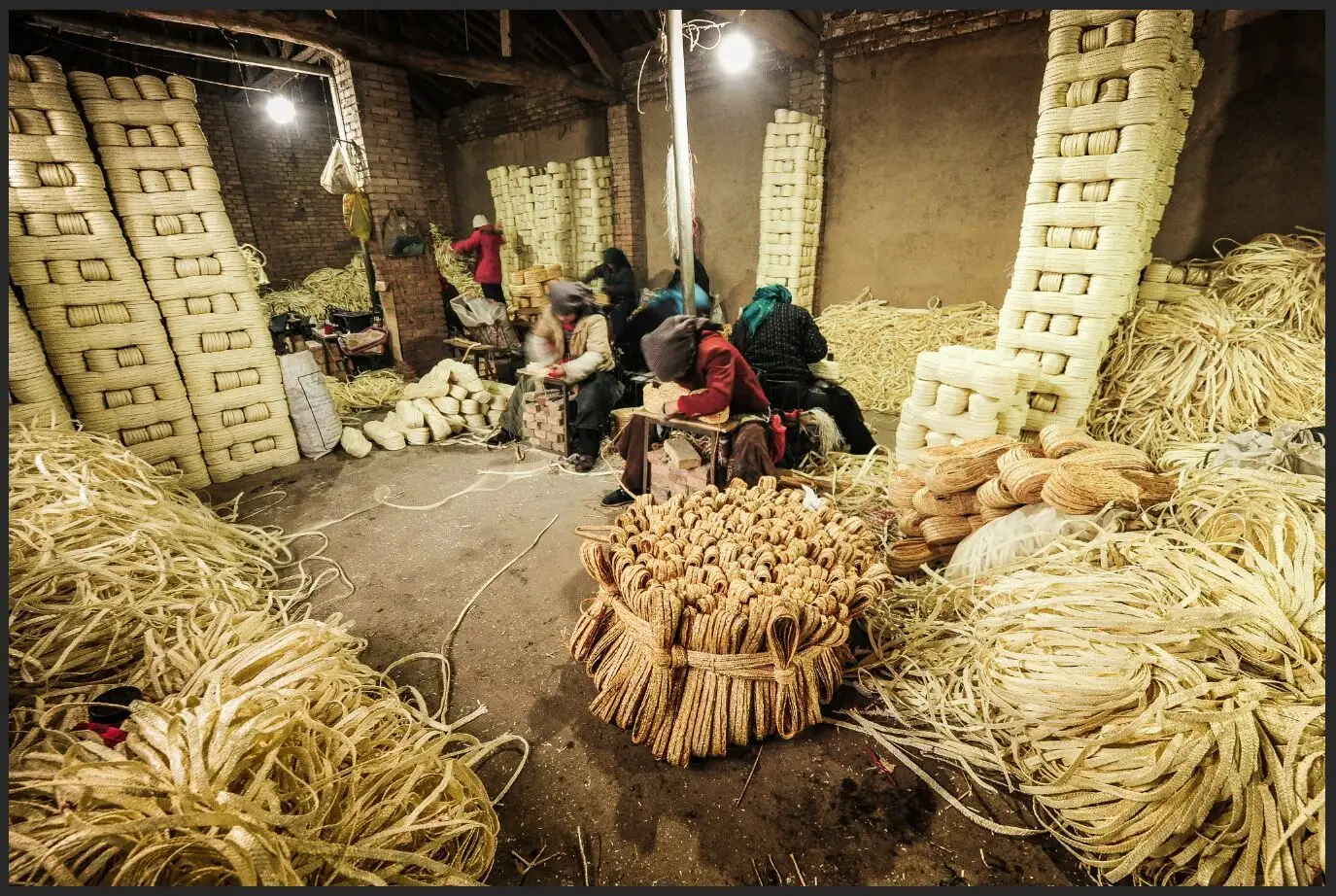
Я вспоминаю один сценарий, когда крупяная фабрика по производству круп перешла с механических датчиков на решение, полностью основанное на ИИ. Результатом стало меньше возвратов продукции из распределительных центров, а также более плавный поток продукции. Операторы сказали мне, что это ощущалось как «полное обновление» их повседневной рутины.
Почему соблюдение требований безопасности пищевых продуктов имеет значение
Соблюдение требований безопасности пищевых продуктов это не просто юридическое требование; это моральная ответственность. Независимо от того, управляете ли вы небольшой пекарней или крупным заводом по переработке пищевых продуктов, обеспечение безопасности каждого продукта для потребления имеет решающее значение. Инструменты ИИ помогают отслеживать чистоту, однородность продукта и точность маркировки.
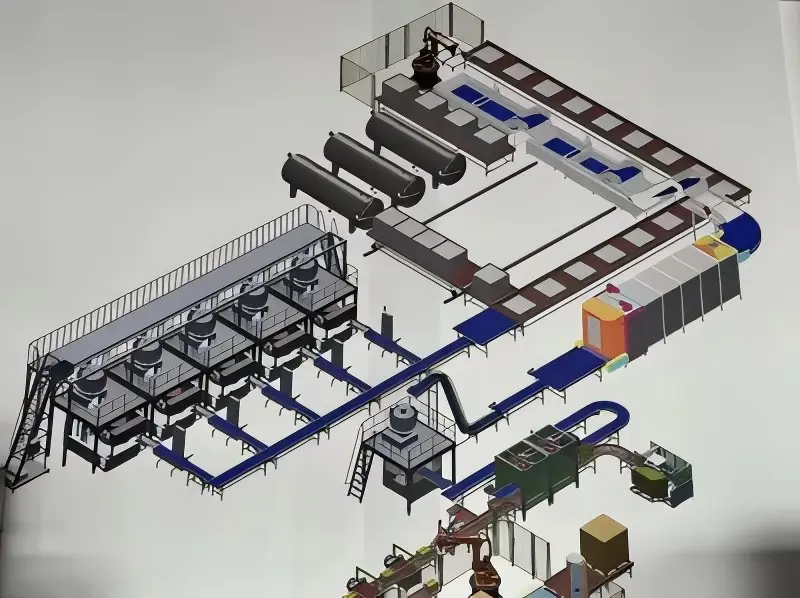
Упаковка ИИ Линии часто содержат расширенные проверки гигиены. Камеры и датчики могут определять загрязняющие вещества, которые проникают в зоны упаковки. Эта система также может проверять правильность маркировки относительно аллергенов, информации о питательной ценности или кодов партий.
«Не подготовившись, вы готовитесь к неудаче» — Бенджамин Франклин
Когда я впервые работал на заводе, сканирование на наличие загрязняющих веществ в основном проводилось путем случайных проверок. Было легко пропустить загрязненную партию, особенно в больших объемах. Технология ИИ минимизирует этот риск, предупреждая персонал в тот момент, когда на конвейерной ленте появляется необычный посторонний предмет. станция отбраковки автоматически направляет подозрительный объект на дальнейший анализ.
Концепция антистатической упаковки
Антистатическая упаковка предназначен для защиты электронных компонентов, чувствительных фармацевтических препаратов и даже деликатных пищевых продуктов от электростатического повреждения. Статическое электричество или небольшой электрический заряд могут разрушить микрочипы или ухудшить некоторые товары, если их не контролировать должным образом. Упаковочные линии с искусственным интеллектом гарантируют, что антистатический слой не поврежден и эффективно запечатан.
На некоторых объектах сухость воздуха или трение от высокоскоростных операций могут вызывать накопление статического электричества. Интеллектуальная упаковочная машина может измерять влажность и температуру, одновременно проверяя, остаются ли защитные покрытия целыми. Как только она замечает необычные скачки электрического заряда, она немедленно вносит коррективы или оповещает оператора.
Я наблюдал за машинами, которые автоматически распыляют мелкий туман или регулируют ионизаторы воздуха в ответ на данные от машинное обучение анализ. Это помогает создать безопасную среду для чувствительных к статическому электричеству продуктов. Сосредоточившись на таком специализированном решении, заводы избегают дорогостоящих отзывов или неисправностей продукта в дальнейшем.
Методы обнаружения дефектов пузырей с использованием ИИ
Проверка на наличие дефектов пузырей фокусируется на прозрачных или полупрозрачных упаковках, которые используются в фармацевтических препаратах, электронике или потребительских товарах. Блистерная упаковка хранит отдельные предметы отдельно и защищает от влаги. Если блистер проколот или неправильно сформирован, продукт может испортиться быстрее или стать опасным, если это лекарство.
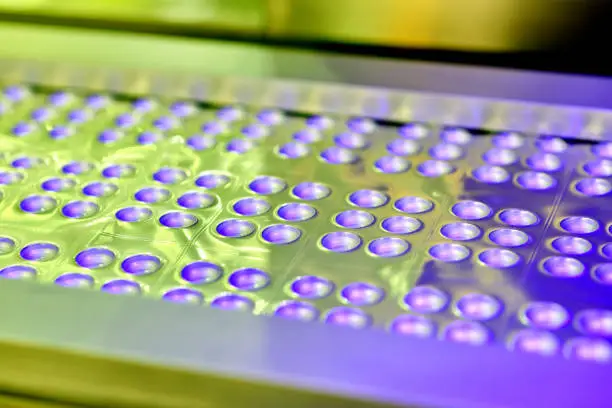
Инспекция с помощью искусственного интеллекта для упаковки пакетов также может применяться здесь. У вас есть камера, сканирующая каждую полость волдыря, чтобы убедиться, что она полностью сформирована и запечатана. машинное обучение аспект включает в себя запоминание нормальной формы волдырей и распознавание даже малейшей деформации.
Существуют две основные стратегии обнаружения:
- Оптическое профилирование:
Камеры смотрят на поверхность, анализируя небольшие ямочки или пузырьки воздуха. Если форма отклоняется на долю миллиметра, срабатывает сигнал тревоги. - Инфракрасное сканирование:
Некоторые передовые линии включают инфракрасные датчики, которые обнаруживают утечки или изменения толщины. Это особенно полезно, если материал блистера включает несколько слоев.
Когда я тестировал эти системы, я понял, что они без проблем справляются с большими объемами — тысячами блистерных упаковок в час. Анализ выполняется автоматически, что экономит часы ручной проверки. Кроме того, данные с каждой смены возвращаются в модель ИИ, что со временем повышает точность обнаружения.
Повышение эффективности с помощью предиктивного обслуживания на основе ИИ
Прогностическое обслуживание использует данные машин в реальном времени для прогнозирования сбоев оборудования до того, как они произойдут. Упаковочная линия имеет много движущихся частей: ремни, шестерни, двигатели, датчики. Со временем износ становится нормальным явлением. Вместо того чтобы ждать очевидной неисправности, системы ИИ отслеживают температуру, вибрацию и другие подсказки, которые могут сигнализировать о будущей поломке.
Например, если уровень вибрации двигателя многократно превышает определенные пороговые значения, система отправляет оповещение. Оператор может запланировать проверку или замену, предотвращая внезапную остановку в часы пик. Такой подход не только экономит деньги, но и обеспечивает большую надежность всего производственного конвейера.
Я видел, как упаковочные цеха принимали этот метод, чтобы сократить незапланированные простои более чем на 40%. Раньше они чинили вещи после того, как они ломались. Теперь они чинят их до того, как они станут большей проблемой. Этот сдвиг является большой частью устойчивость движение тоже. Профилактика часто приводит к снижению потребления энергии и уменьшению количества потраченных впустую ресурсов.
Повышение эффективности с помощью предиктивного обслуживания на основе ИИ
Прогностическое обслуживание использует данные машин в реальном времени для прогнозирования сбоев оборудования до того, как они произойдут. Упаковочная линия имеет много движущихся частей: ремни, шестерни, двигатели, датчики. Со временем износ становится нормальным явлением. Вместо того чтобы ждать очевидной неисправности, системы ИИ отслеживают температуру, вибрацию и другие подсказки, которые могут сигнализировать о будущей поломке.
Например, если уровень вибрации двигателя многократно превышает определенные пороговые значения, система отправляет оповещение. Оператор может запланировать проверку или замену, предотвращая внезапную остановку в часы пик. Такой подход не только экономит деньги, но и обеспечивает большую надежность всего производственного конвейера.
Я видел, как упаковочные цеха принимали этот метод, чтобы сократить незапланированные простои более чем на 40%. Раньше они чинили вещи после того, как они ломались. Теперь они чинят их до того, как они станут большей проблемой. Этот сдвиг является большой частью устойчивость движение тоже. Профилактика часто приводит к снижению потребления энергии и уменьшению количества потраченных впустую ресурсов.
Лучшие практики для бесшовной интеграции ИИ
Внедрение искусственного интеллекта в производственную линию может показаться сложным. На самом деле, это проще, чем думают большинство людей, если следовать лучшим практикам:
- Пилотные проекты:
Начните с небольшого участка линии. Может быть, сосредоточьтесь только на антистатическая упаковка или проверки этикеток. Оцените производительность, соберите данные и используйте их для тонкой настройки модели ИИ. - Обучение сотрудников:
Некоторые работники могут беспокоиться о новых технологиях. Покажите им, как они упрощают их задачи, а не делают их избыточными. Когда я курировал внедрение нового ИИ, сотрудники наслаждались более плавным рабочим процессом и более простым устранением неполадок. - Безопасность данных и соответствие требованиям:
Хранение производственных данных — это здорово, но держите их в безопасности. Обязательно следуйте местным правилам конфиденциальности, если речь идет о какой-либо личной информации. - Постоянная обратная связь:
Пусть система ИИ учится на ошибках. Если она помечает слишком много элементов для отклонения, отрегулируйте пороговые уровни или маркируйте данные более точно.
Эти шаги в сочетании с открытым общением могут обеспечить переход от механических датчиков к датчикам на основе искусственного интеллекта. встроенная инспекция ветерок. Я провел несколько линий через это изменение, и каждый раз общий моральный дух улучшался, когда люди видели ощутимые преимущества.
Распространенные проблемы и практические решения
Ни одна система не идеальна. Упаковочные линии с искусственным интеллектом иногда сталкиваются с проблемами. Знание этих проблем может помочь вам решить их немедленно.
1. Перегрузка данными
Сбор миллионов точек данных в час — это замечательно, но это может подавить неподготовленный персонал. Как исправить? Используйте удобные панели мониторинга и ограничьте метрики теми, которые имеют практическое значение. Извлекайте большие данные в ежедневные или еженедельные аналитические данные для более простого принятия решений.
2. Первоначальные затраты
Да, новое оборудование и обучение ИИ могут быть дорогими. Однако окупаемость инвестиций через сокращение отходов, лучше соблюдение безопасности пищевых продуктов, и меньшее количество отзывов обычно уравновешивает чашу весов. Поговорите с поставщиками о финансировании или поэтапных подходах. Внедряйте ИИ поэтапно, а не делайте полную перестройку.
3. Совместимость оборудования
Иногда старые машины не имеют датчиков или цифровых выходов для интеграции с ИИ. Не всегда необходимо покупать совершенно новые линии. Модернизация или частичная модернизация могут помочь вам объединить старые системы с новыми технологиями. Однажды мы отремонтировали 10-летнюю линию, добавив датчики камеры и небольшой компьютер для обработки данных.
4. Текущее обслуживание
Даже самое умное устройство требует ухода. профилактическое обслуживание проверки, держите камеры в чистоте и регулярно обновляйте программное обеспечение. Повторно калибруйте датчики по установленному графику, чтобы убедиться, что ваши показания остаются точными.
Я лично помогал модернизировать старые линии. Самый большой вывод заключается в том, что небольшие изменения могут привести к серьезным улучшениям. Например, обновление камер или добавление расширенного программного слоя часто приводит к огромному скачку в согласованности упаковки.